---- A.1 MITOS12
---- A.2 GlaS 2015
---- A.3 Janowczyk's epithelium dataset
---- A.4 Gleason 2019
---- A.5 MoNuSAC 2020
---- A.6 Artefact dataset
---- References
A. Description of the datasets
Several datasets, mostly coming from digital pathology competitions, are used throughout this thesis for our experiments and analysis. We provide here a description of their main characteristics, for reference.
A.1 MITOS12
- General information: Dataset of the MITOS12 mitosis detection challenge (post-challenge publication by Roux et al. [1]), organized by IPAL Laboratory, TRIBVN Company, Pitié-Salpêtrière Hospital and CIALAB and hosted at ICPR 2012.
- Website: http://ludo17.free.fr/mitos_2012/index.html
- Availability: Training and test set images and annotations available.
- Main characteristics: Patches from H&E-stained slides (each patch corresponding to a 512x512µm² region) extracted from breast cancer biopsies. 3 different acquisition devices: Aperio & Hamamatsu RGB scanners + multispectral microscope (only Aperio & Hamamatsu are used in our experiments), with resolutions of 0.25, 0.23 and 0.19 µm/px, respectively (corresponding to 40x magnification). Number of samples: 5 patients, 50 patches.
- Training set: 35 images from all 5 patients, containing 226 mitosis.
- Test set: 15 images from all 5 patients, containing 103 mitosis.
- Annotations: Single pathologist, segmented mitotic nuclei. See Figure A.1 for an illustration of the acquisition and annotation pipeline.
- Balance: The mitosis regions occupy 0.09% of the total tissue area. There is an average of 6.6 mitosis per 2048x2048px image patch.
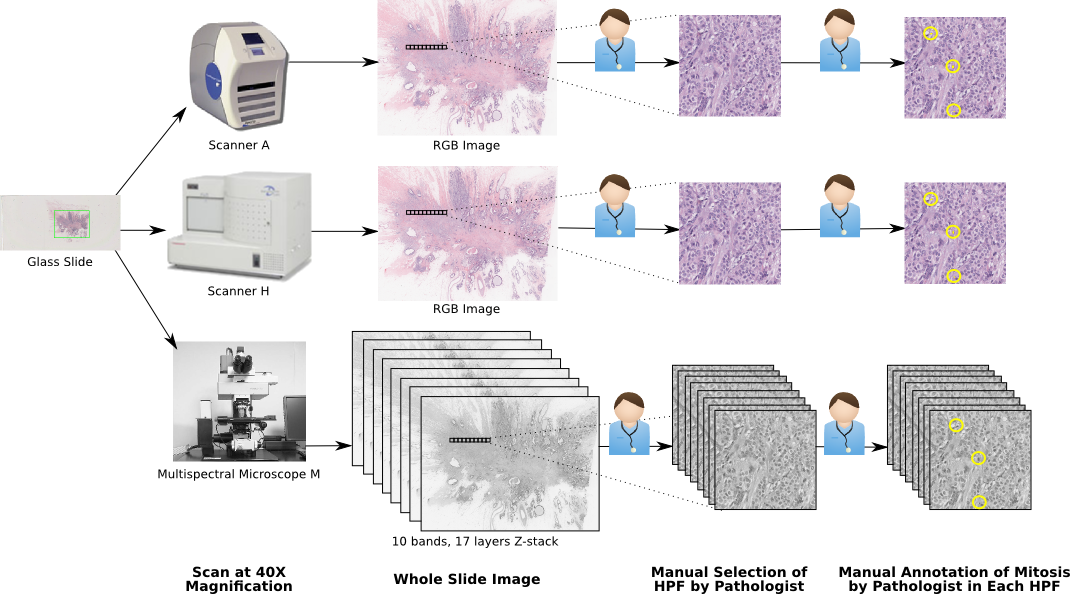
A.2 GlaS 2015
- General information: Dataset of the GlaS 2015 gland segmentation challenge (post-challenge publication by Sirinukunwattana et al. [2]), organized by Bioimage Analysis Lab (University of Warwick), Qatar University, Eindhoven University of Technology & UMC Utrecht, and University Hospital of Coventry and Warwickshire and hosted at MICCAI 2015.
- Website: https://warwick.ac.uk/fac/cross_fac/tia/data/glascontest/
- Availability: Training and test set images and annotations available.
- Main characteristics: Patches from H&E-stained slides extracted from colorectal cancer tissue. Acquisition device: Zeiss scanner, resolution of 0.62 µm/px (corresponding to 20x magnification). Number of samples: 16 patients, 165 patches.
- Training set: 85 images from all 16 patients (but from different “visual fields” than those in the test set). 37 images come from “benign” tissue, 48 from “malignant” tissue.
- Test set: 80 images from all 16 patients. Further split in test set A (33 benign, 27 malignant) and B (4 benign, 16 malignant). In the challenge, test A was used for “off-site” testing, and test B for “on-site” testing on the day of the challenge event.
- Annotations: Single pathologist, segmented glands. See Figure A.2 for examples of images and annotations from the training set.
- Balance: Training set: 769 glands making up 50% of the total pixel area. Test set: 761 glands making up 51% of the total pixel area.
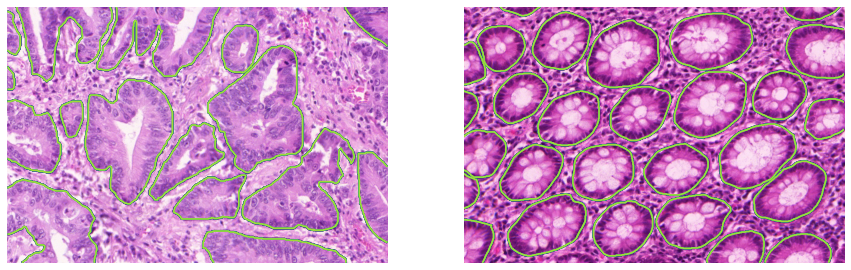
A.3 Janowczyk’s epithelium dataset
- General information: Dataset accompanying the “Deep learning for digital pathology image analysis” tutorial by Janowczyk and Madabhushi [3] for the epithelium segmentation task.
- Website http://www.andrewjanowczyk.com/deep-learning/
- Availability Images and annotations available.
- Main characteristics: Patches from H&E-stained slides extracted from oestrogen receptor positive (ER+) breast cancer tissue. Acquisition device: unspecified, 20x magnification. Number of samples: 42 patients, 42 patches.
- Annotations: Single pathologist, segmented epithelium region (see Figure A.3).
- Balance The epithelium regions occupy 33.5% of the total pixel area
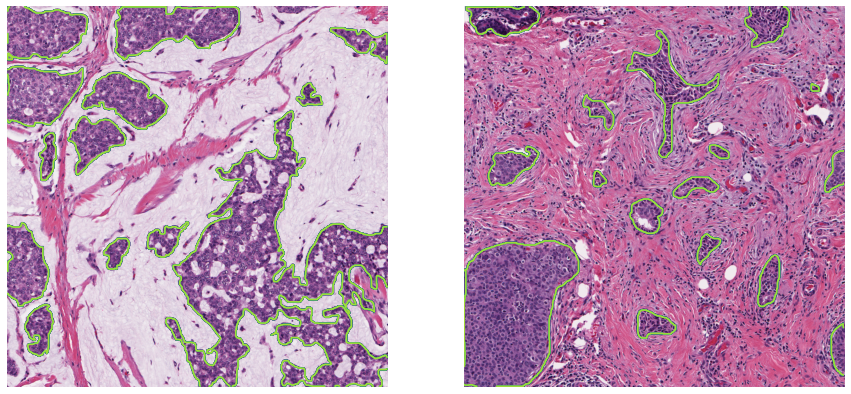
A.4 Gleason 2019
- General information: Dataset of the Gleason 2019 Gleason pattern segmentation and grading challenge, organised by the University of British Columbia with data from the Vancouver Prostate Center, and hosted at MICCAI 2019. No post-challenge publication has been made by the organisers. A 2022 publication from the winning team is available in Qiu et al. [4], and several publications have been made by the organizing team using the data [5]–[8].
- Website: https://gleason2019.grand-challenge.org/
- Availability Training set images and individual expert annotations, test set images.
- Main characteristics TMA cores from H&E-stained prostate cancer tissue. Acquisition device: SCN400 Slide Scanner, 40x magnification. Number of samples: 331 cores from around 230 patients (the number of cores in the available data doesn’t exactly match the numbers reported in the different publications).
- Training set: 244 cores.
- Test set: 87 cores.
- Annotations: Individual annotations from 6 different pathologists (not all pathologists annotated all slides).
- Balance: Large imbalance between the different grades, with Gleason pattern 5 very sparsely represented.
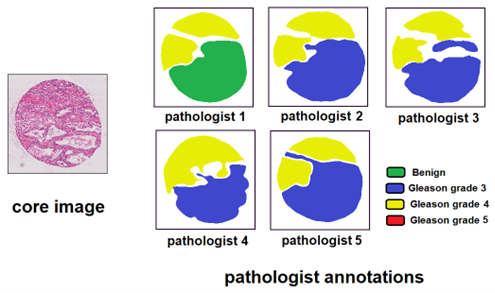
A.5 MoNuSAC 2020
- General information: Dataset of the MoNuSAC 2020 nuclei instance segmentation and classification challenge, organised by the Case Western Reserve University in Cleveland, Ohio, and the Indian Institute of Technology in Bombay, India, and hosted at ISBI 2020. A post-challenge publication was made by Verma et al. [9]. We published a comment article noting some errors in the challenge results [10], leading to a response and partial correction of the results from Verma et al. [11].
- Website https://monusac-2020.grand-challenge.org
- Availability Training and test set images and annotations available. Predictions of four of the top-five teams on the test set are also available. Source code for reading the annotation file and for the implementation of the evaluation metric available on GitHub1.
- Main characteristics: Patches from H&E-stained tissue from four different organs (lung, prostate, kidney and breast) extracted from the TCGA portal at 40x magnification. Number of samples: 71 patients, 310 patches (with more than 45.000 annotated nuclei).
- Training set: 46 patients, 209 patches.
- Test set: 25 patients, 101 patches.
- Annotations: Single annotation per patch available. Annotations were made by “engineering graduate students” with quality control by “an expert pathologist” [9].
- Balance: Large class imbalance, with 20-30x more epithelial and lymphocyte nuclei than macrophages and neutrophils. However, the latter are much larger, so that the “per-pixel” imbalance is less strong.
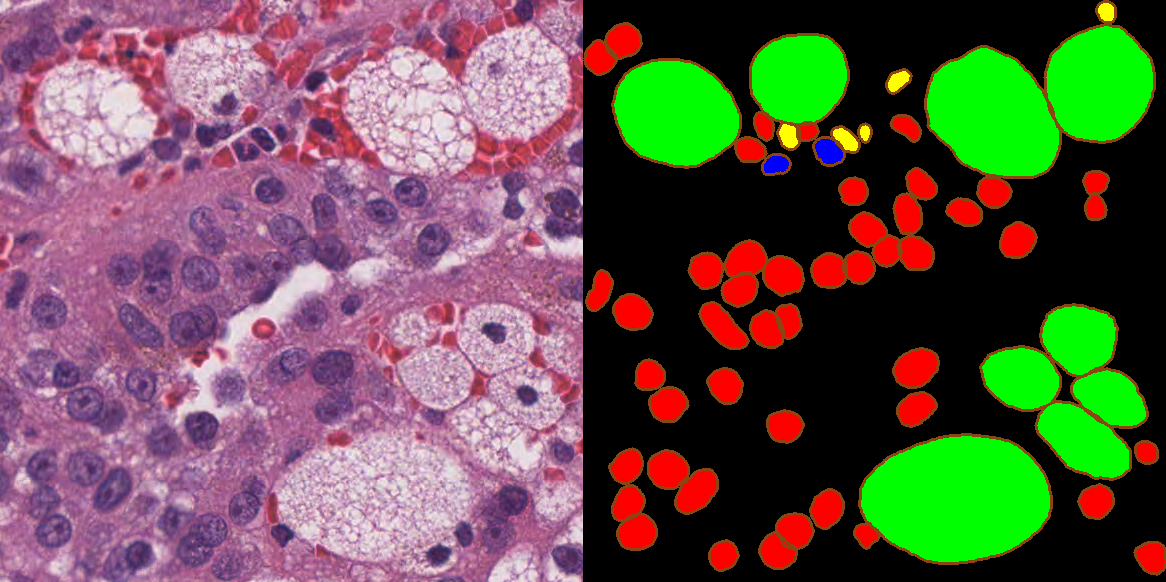
A.6 Artefact dataset
- General information Dataset used in several of our publications [12], [13] on artefact segmentation in digital pathology.
- Website https://doi.org/10.5281/zenodo.3773097
- Availability Low-resolution (1.25x and 2.5x magnification) WSIs and annotation masks, as well as some extracted patches with patch-level annotations on the type of artefacts present.
- Main characteristics A total of 22 WSIs from 3 different tissue blocks. Block A (20 slides): 10 H&E-stained and 10 IHC (anti-pan-cytokeratin) from colorectal cancer tissue. Block B (1 slide): IHC (anti-pan-cytokeratin) from gastroesophageal junction (dysplasic) lesion. Block C (1 slide): IHC (anti-NR2F2) from head and neck carcinoma.
- Training set: 18 slides from block A.
- Validation set: 2 slides from block A + 1 slide from block B, as well as 21 image patches extracted from those 3 slides.
- Test set: 1 slide from block C.
- (Additionally in our 2020 publication [13], 4 slides from TCGA are used for a qualitative assessment)
- Annotations: Very rough annotations made by A. Foucart for block A and B, more precise annotations made by an expert technologist for block C. All types of artefactual regions are segmented (including tissue folds and tears, ink artefacts, pen markings, blur, etc.). A total of 918 distinct artefacts are annotated in the training set.
- Balance: Very low density of annotated objects (2% positive pixels in the training set, 8% in the validation set and 9% in the test slide).
![Figure A.6. Annotated slide from the artefact training set, with imprecise delineation and many unlabelled artefacts, including blurry regions and smaller tears. Image reproduced from [13].](./fig/A-6.jpg)
References
[1] L. Roux et al., “Mitosis detection in breast cancer histological images An ICPR 2012 contest,” Journal of Pathology Informatics, vol. 4, no. 1, p. 8, 2013, doi: 10.4103/2153-3539.112693.
[2] K. Sirinukunwattana, J. P. W. Pluim, H. Chen, and Others, “Gland segmentation in colon histology images: The glas challenge contest,” Medical Image Analysis, vol. 35, pp. 489–502, 2017, doi: 10.1016/j.media.2016.08.008.
[3] A. Janowczyk and A. Madabhushi, “Deep learning for digital pathology image analysis: A comprehensive tutorial with selected use cases,” Journal of Pathology Informatics, vol. 7, no. 1, 2016, doi: 10.4103/2153-3539.186902.
[4] Y. Qiu et al., “Automatic Prostate Gleason Grading Using Pyramid Semantic Parsing Network in Digital Histopathology,” Frontiers in Oncology, vol. 12, Apr. 2022, doi: 10.3389/fonc.2022.772403.
[5] D. Karimi, G. Nir, L. Fazli, P. C. Black, L. Goldenberg, and S. E. Salcudean, “Deep Learning-Based Gleason Grading of Prostate Cancer from Histopathology Images - Role of Multiscale Decision Aggregation and Data Augmentation,” IEEE Journal of Biomedical and Health Informatics, vol. 24, no. 5, pp. 1413–1426, 2020, doi: 10.1109/JBHI.2019.2944643.
[6] D. Karimi, G. Nir, L. Fazli, P. C. Black, L. Goldenberg, and S. E. Salcudean, “Deep Learning-Based Gleason Grading of Prostate Cancer From Histopathology Images—Role of Multiscale Decision Aggregation and Data Augmentation,” IEEE Journal of Biomedical and Health Informatics, vol. 24, no. 5, pp. 1413–1426, May 2020, doi: 10.1109/JBHI.2019.2944643.
[7] G. Nir et al., “Comparison of Artificial Intelligence Techniques to Evaluate Performance of a Classifier for Automatic Grading of Prostate Cancer From Digitized Histopathologic Images,” JAMA Network Open, vol. 2, no. 3, p. e190442, Mar. 2019, doi: 10.1001/jamanetworkopen.2019.0442.
[8] G. Nir et al., “Automatic grading of prostate cancer in digitized histopathology images: Learning from multiple experts,” Medical Image Analysis, vol. 50, pp. 167–180, Dec. 2018, doi: 10.1016/j.media.2018.09.005.
[9] R. Verma et al., “MoNuSAC2020: A Multi-Organ Nuclei Segmentation and Classification Challenge,” IEEE Transactions on Medical Imaging, vol. 40, no. 12, pp. 3413–3423, Dec. 2021, doi: 10.1109/TMI.2021.3085712.
[10] A. Foucart, O. Debeir, and C. Decaestecker, “Comments on ‘MoNuSAC2020: A Multi-Organ Nuclei Segmentation and Classification Challenge,’” IEEE Transactions on Medical Imaging, vol. 41, no. 4, pp. 997–999, Apr. 2022, doi: 10.1109/TMI.2022.3156023.
[11] R. Verma, N. Kumar, A. Patil, N. C. Kurian, S. Rane, and A. Sethi, “Author’s Reply to ‘MoNuSAC2020: A Multi-Organ Nuclei Segmentation and Classification Challenge,’” IEEE Transactions on Medical Imaging, vol. 41, no. 4, pp. 1000–1003, Apr. 2022, doi: 10.1109/TMI.2022.3157048.
[12] A. Foucart, O. Debeir, and C. Decaestecker, “Artifact Identification in Digital Pathology from Weak and Noisy Supervision with Deep Residual Networks,” in 2018 4th International Conference on Cloud Computing Technologies and Applications (Cloudtech), Nov. 2018, pp. 1–6. doi: 10.1109/CloudTech.2018.8713350.
[13] A. Foucart, O. Debeir, and C. Decaestecker, “Snow Supervision in Digital Pathology: Managing Imperfect Annotations for Segmentation in Deep Learning,” 2020, doi: 10.21203/rs.3.rs-116512.